Quadrotor Fault Tolerant Flight Control
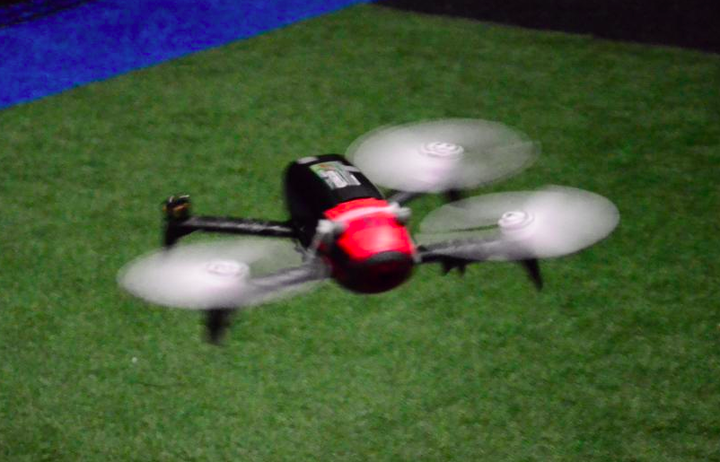
As the most commonly used and simplest multi-rotor drones, quadrotors are especially vulnerable to rotor failures because they lack rotor redundancy. This project aims to introduce novel flight control methods to mitigate the consequence of in-flight motor failures, which rarely happen but are still a big safety threat after large-scale deployment of drones in the future.
To be specific, the project leverages the incremental nonlinear dynamic inversion (INDI) control method, to stabilize and control a quadrotor with failure of a single rotor or two opposing rotors, under significant aerodynamic disturbances. These strong aerodynamic effects are dominant in high-speed flight, or windy conditions. Thanks to the robustness of the INDI controller, the damaged quadrotor maintains controllable in spite of these aeordynamic disturbances.
We have validated the proposed controllers in a large-scale wind tunnel, named Open Jet Facility at TU Delft. Despite a complete loss of one or two propellers, the tested quadrotor can maintain high-speed flight. In addition to validating the controller, these wind tunnel flight tests have produced valuable data for identifying aerodynamic models of a quadrotor, in both nominal and damaged conditions.
Another focus of the research aims at recovering the damaged quadrotor after motor failure. When a motor failure is detected, a quadrotor might have entered a detrimental flight condition, such as an upside-down orientation, where the drone can hardly recover to stable flights using the state-of-art methods. Therefore, we have developed an upset recovery controller, using a novel control allocation approach. We have explored the performance of using nonlienar model predictive controller (NMPC) on the upset recovery task. The fail-safe performance is mind-blowing. It is able to recover the quadrotor after motor failure not only from extreme orientations, but also from agile flights.
Above research, all rely on external sensors to provide reliable state estimates of the damaged quadrotor. This is a strong assumption in real-world scenarios where motion capture systems are not available and GPS signals can be degraded. For this reason, we have explored using onboard vision sensors to provide a full-state estimation of the drone with motor failures. The high-speed yaw motion makes it challenging to use a vision-based estimator as it brings motion blur. Hence we have conducted a research project to explore the potential of using standard frame-based cameras and event-based cameras on quadrotor fail-safe tasks. This work has been awarded a Best Paper Award from IEEE Robotics and Automation Letters in 2021.